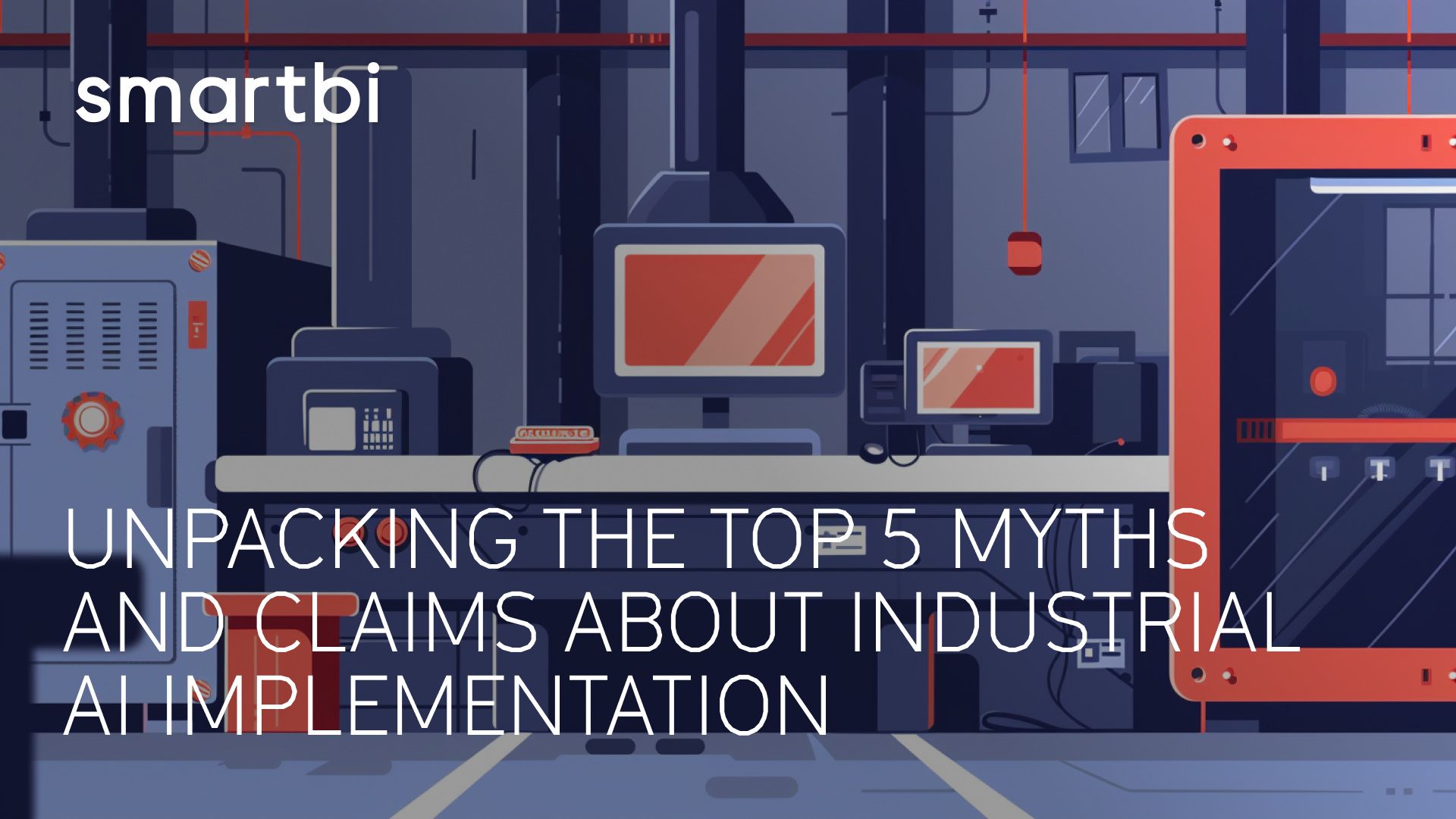
Misconceptions surrounding the use of industrial artificial intelligence are growing at the same speed as the number of AI product announcements. In this blog post, we aim to challenge common myths and ideas, clarify beliefs about AI adoption, and provide alternative perspectives on the topics.
Claim 1: “AI equals generative AI.”
This isn't true. While generative artificial intelligence (GenAI) is the most spoken-of use case, it represents merely a fraction of AI's capabilities. AI's landscape is full of technologies, from data analysis to predictive modeling, image recognition, natural language processing, computer vision, and robotics; and each can add value to different business and operation functions.
"Traditional AI" is often perceived as less exciting (we didn't want to use the word 'boring'). For instance, machine learning (ML) tends to work in pairs with GenAI in the solutions we build. We use ML to analyze manufacturers' data and make predictions while GenAI creates reports and suggests improvements to scheduling operations. By adding "traditional AI" to software and devices, industrial companies can often improve production processes' efficiency, predict equipment maintenance needs, optimize supply chains, and reduce the waste of materials and tools.
Claim 2: "With paid subscriptions to commercial LLM models, our data is protected and won't be used externally.”
Not quite true. The most widely used and popular LLMs, such as GPT, Gemini, Claude, and Falcon, generally process data through cloud-based services. While there are options to ensure that a company's data isn't used for training the model, the data processed by these models is often handled on the providers' servers. This approach has significant data privacy concerns, so we don't recommend using it, especially in industries such as healthcare, financial services, telecommunications, chemical manufacturing, energy production, and heavy machinery, where rigorous privacy measures are crucial.
Excellent, secure alternatives are available through open-source solutions such as Llama 2, GPT-J-6b, and GPT-NeoX-20 B. These can be deployed locally, enabling advanced AI without transmitting data to external servers and ensuring safe data management. While they require additional, often hefty hardware, this investment makes sense for businesses prioritizing safety. Not surprisingly, many of our clients choose this secure option to improve their operations and production.
Claim 3: "AI solutions are expensive."
True, but misleading. While initial costs can be high, the return on investment often justifies the expense. The cost varies based on the solution's complexity, whether it's adding machine learning algorithms, implementing analytics, or building a computer vision system from scratch. While initial investments in AI solutions can be high, their value and efficiency improvements often quickly pay for themselves. For instance, employing AI to improve safety procedures in a bigger factory can save millions of euros annually. Another example: One of our clients reduced a seven-week inspection period to just three days with the help of AI. Needless to say, they were very happy with the new, streamlined inspection operations.
Claim 4: "We don't need extensive strategic planning before jumping into AI implementation."
Not quite. Diving into AI isn't just about the technology, stacks, and data architectures. A comprehensive vision is essential. You're setting the stage for how your organization evolves and grows, including business practices, culture, and even future employment. Your future successes are tied to what you build today with the help of AI. Implementing AI requires an understanding of how the business side of things integrates with the technological aspects.
A well-rounded AI agency can provide the necessary technical expertise paired with industrial business knowledge. At Smartbi, we have expertise in solving problems for large industrial companies operating in sectors such as manufacturing, forestry, chemistry, and others. When we offer technical expertise, it comes with a focus on the big picture – how the solutions can either add to your bottom line or save time in critical operations.
Claim 5: "We don’t need standalone AI solutions – we are waiting for an AI update to be integrated into our existing programs.”
This is debatable as relying solely on incremental AI updates is often risky and fails to address an organization’s broader needs. Industrial programs that are not AI-first often fail to integrate the advanced capabilities that AI can provide, leading to missed opportunities. We encourage organizations to plan AI strategies parallel to their existing operations. By doing so, they can ensure they start reaping the benefits of the latest technology and, most importantly, gain a competitive advantage in an increasingly competitive global market.
Get in touchCould AI be applied to your business case?
Subscribe to the newsletter and learn how AI can solve business challenges.