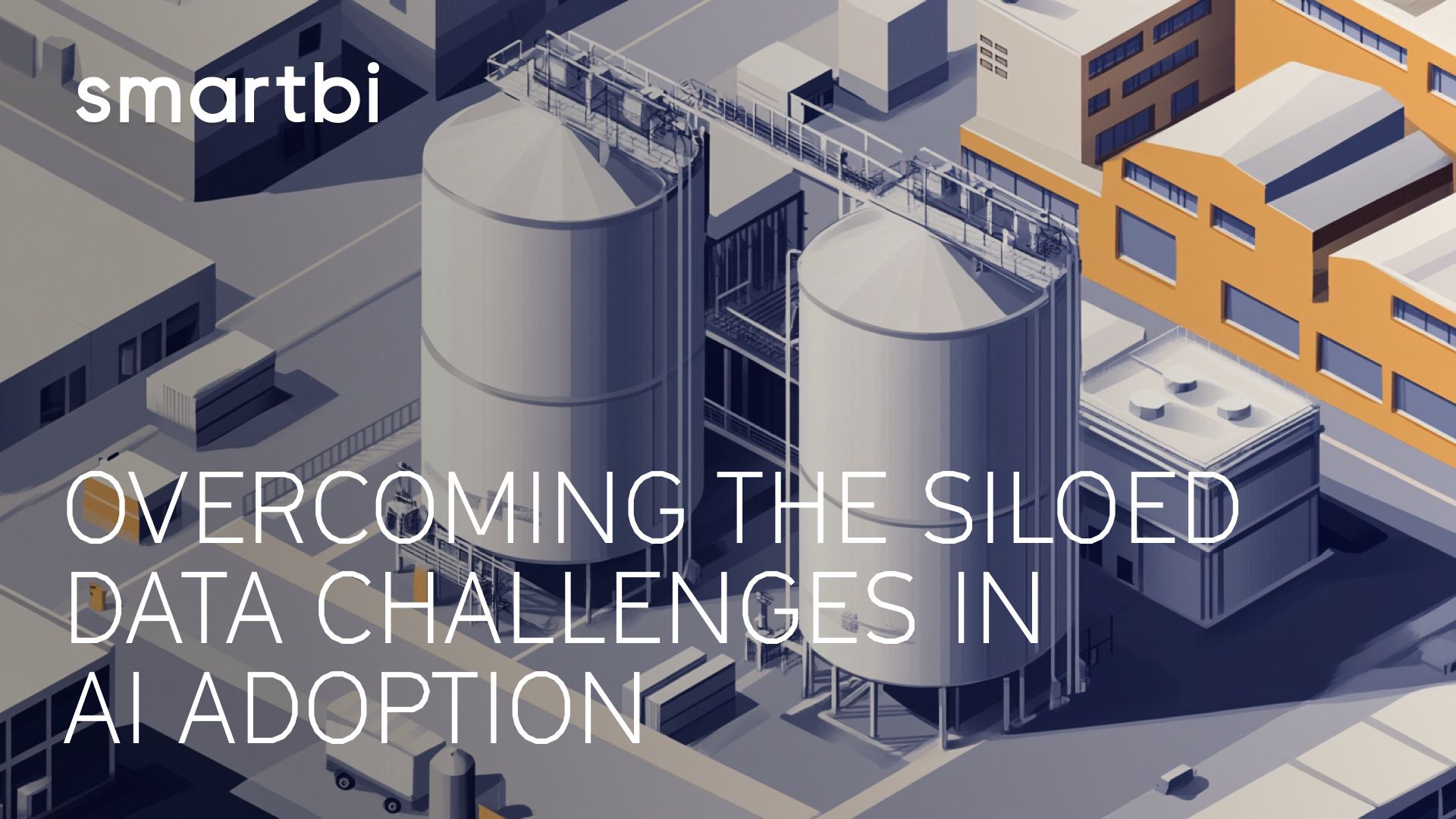
The foundation of AI: Why data quality matters
High-quality data is the bedrock upon which successful AI models are built. It's not merely about the quantity of information; it's about having the correct information from the right places – or, as in industrial settings, the right machines and types of equipment.
Diverse, representative data ensures that AI models make accurate and relevant decisions. When trained on insufficient data, AI systems can produce skewed results, leading to errors in production and hindering operations.
The hurdle: The "trapped" data
Here's a hard truth: most organizations don't have clean, AI-ready datasets just waiting to be implemented. Data often resides in silos, is incomplete, or is riddled with errors. In many factories, critical data is "trapped" in machines, inaccessible even to the company's employees.
Sometimes, data is not just "trapped" but deliberately siloed. In industries like manufacturing and energy, data is not just valuable – it's often highly confidential. When data contains personal or sensitive operational information, companies are (understandably) hesitant to make it accessible.
This messy reality is the leading challenge we have witnessed when implementing AI solutions in industrial settings.
The solution: Bridging the gap between operational technology (OT) and information technology (IT)
One of the most promising signs of utilizing data has been the growing integration of Operational Technology (OT) and Information Technology (IT). Traditionally, these have been separate domains, each with its focus and expertise. Operational Technology covers the hardware and machinery, such as the operation of manufacturing equipment, the optimization of supply chains, and the maintenance of industrial plants. Information Technology, on the other hand, involves the use of digital systems for data management, networking, cybersecurity, and software development.
The power of OT and IT joining forces
Closing the division between operational data and IT systems
Example: A manufacturing plant integrates its production line sensors with the company's central data warehouse. Real-time data on machine production rates is now directly accessible to data analysts – and for more developed companies, AI models – enabling automated inventory management based on production rates.
Same data security measures for both operational and analytical needs
Example: A power utility company implements a unified security protocol that allows safe data sharing between its operational technology (OT) systems and IT analytics platforms. This enables AI-driven grid optimization while maintaining strict controls on sensitive infrastructure data.
Facilitating AI solutions aligned with operational challenges
Example: A steel manufacturing plant develops a predictive maintenance program. Instead of relying on fixed schedules or keeping your staff in "reactive mode," alerting them when something fails, AI analyses sensor data to forecast when machines need maintenance. This reduces downtime, extends equipment lifespan, and ensures efficient operations.
Integrating OT and IT represents a crucial step in overcoming the data challenges that have slowed AI adoption in industrial settings. The question is no longer whether to adopt AI but how quickly organizations can overcome these data hurdles to harness the data power effectively.
Get in touchCould AI be applied to your business case?
Subscribe to the newsletter and learn how AI can solve business challenges.