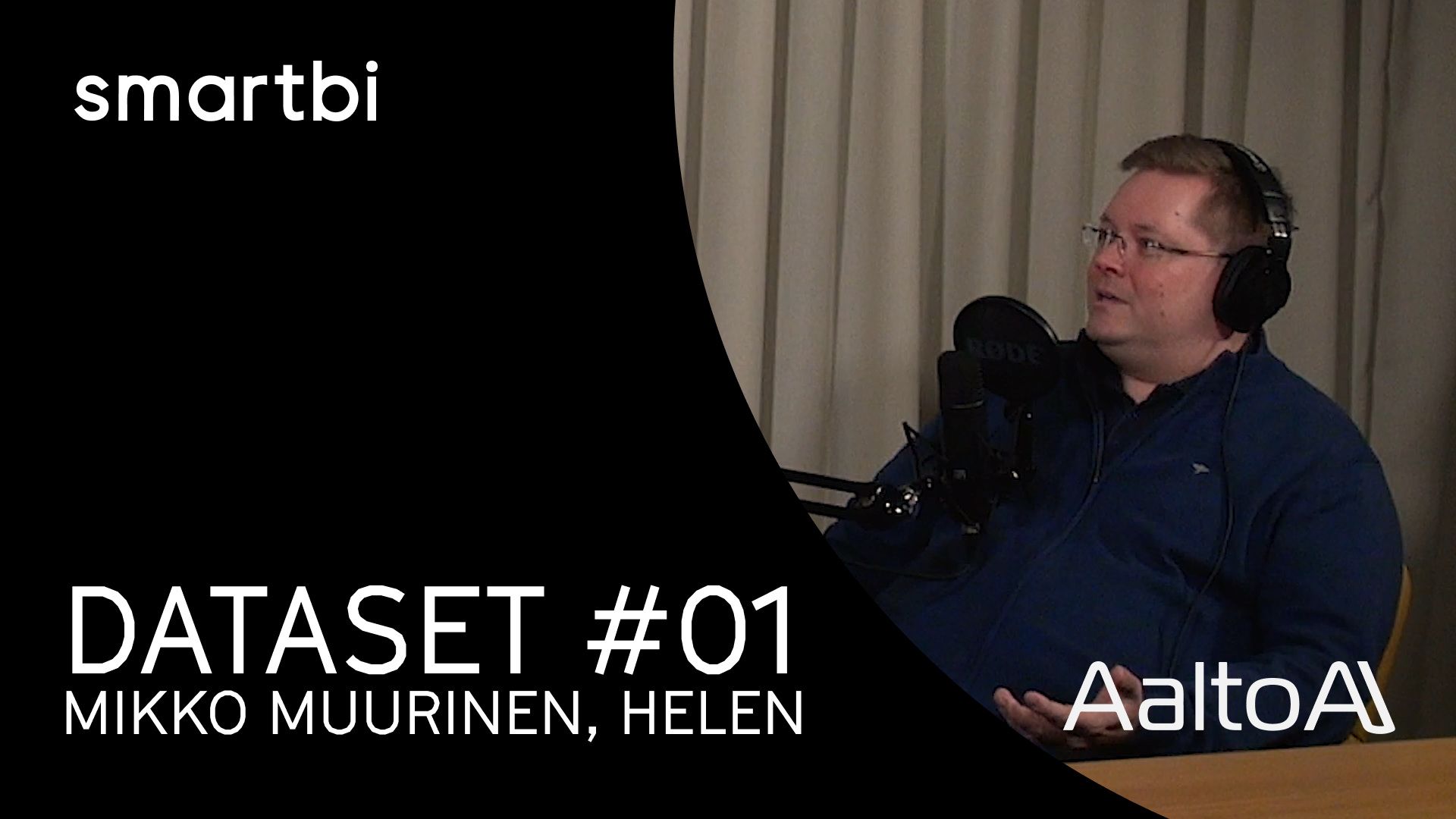
Welcome to the first episode of the Dataset by AaltoAI & Smartbi podcast!
In this episode, we explore the latest AI news and interview Mikko Muurinen, the Head of Data and AI at Helen, one of Finland's largest companies offering electricity and new energy solutions. Mikko discusses how Helen prepares for an AI-driven future and how employees are trained in data and AI. As a bonus for our listeners, we simplify and explain a complex but useful AI-related concept.
Mikko Muurinen and his AI and data teams at Helen are rethinking traditional approaches in the electricity and energy sectors. By integrating AI and data analytics, Helen is modernizing how energy is managed and distributed. Additionally, Mikko will share how energy companies train their employees for an AI-driven future.
Listen to the full episode on Spotify, and for those seeking a quick summary, read the highlights and our discussion with Mikko Muurinen below.
Latest AI news
EU AI Act: The recently published EU Act aims to regulate AI within the EU. It categorizes regulations into four risk-based segments: unacceptable risk, high risk, limited risk, and minimal risk. The goal is to balance the promotion of innovation with safety and ethical considerations. Critics argue that the act may hinder rather than support these objectives.
Claude-3 vs. GPT-4: Antropic has recently unveiled Claude-3, claiming it surpasses OpenAI's GPT-4 performance. Independent evaluations offer a mixed perspective. Claude-3 appears to have improved logical reasoning capabilities but is still prone to errors or hallucinations. Its effectiveness in industrial applications relative to GPT-4 remains to be fully assessed.
Stable Diffusion Version 3: Stability AI announced Stable Diffusion Version 3 in February, showcasing remarkable text-to-image generation capabilities. However, this and previous versions of Stable Diffusion have been controversial due to the non-release of training datasets, sparking debates about the model's co-called openness. Without the datasets, recreating the model from scratch is impossible.
Google DeepMind’s SIMA: The Dataset team had a glimpse of Google's work at DeepMind and their new SIMA model. This generalist AI for 3D virtual environments has been trained to play video games like human players. Unlike its predecessors, which focused on strategy games like chess and Go, the CMI model uses computer vision to understand the environment and interacts with keyboard and mouse inputs. This enables the AI to navigate and play in open-world video games with natural language prompts, showing AI's ability to tackle complex, unstructured environments.
Devin, AI Software Engineer: Developed by Cognition Labs, Devin is an AI software engineer with impressive programming and software development capabilities. It has achieved state-of-the-art results on the Swg Bench, marking a significant performance leap without human instruction. Equipped with tools similar to those used by junior software engineers, including a shell, a code editor, and a browser, Devin can access resources like Stack Overflow to assist in coding tasks.
Mikko Muurinen and Helen – Rethinking traditional approaches in the electricity and energy industry
Mikko leads four teams of over 30 specialists in data science, data governance, data engineering, and digital twin at Helen, transforming traditional energy management, digital customer service, and internal operations.
Helen's AI projects are categorized into classical and generative AI, employing both in-house developed and vendor-supplied solutions.
Helen, a company of roughly 780 people, is currently transitioning from traditional energy production methods to renewable and more sustainable sources. This shift requires integrating real-time data and predictive models to manage the unpredictability of renewable energy sources effectively. One notable project as such is Helen Flex, an IoT solution optimizing energy distribution and storage.
Internally, Helen supports its data-driven culture through educational programs, like the Data Academy, designed to improve employee data literacy (at the moment, 75% of all Helen employees have gone through at least one course in Data Academy).
Simplifying AI Concept: RAG vs. Long Context Models
Imagine you're asking a highly knowledgeable friend a question, but instead of answering from memory, they look up the most accurate, up-to-date information before replying. That's the essence of Retrieval Augmented Generation (RAG).
RAG enhances how AI responds by fetching data from a vast library of information, ensuring the answer is not just smart, but also correct and reliable. RAG was developed to tackle issues like outdated or incorrect information that AI might use to answer questions.
Now, let's contrast this with Long Context Window Models. These AI models are like super-readers, capable of understanding and remembering vast amounts of text at once. With recent advancements, such models can digest entire libraries in a single go, making it easier for them to provide answers without needing to fetch external data.
So, why use RAG when we have these super-readers? The choice depends on the task at hand. RAG offers accuracy and reliability by verifying information against external sources, making it great for fact-based queries. Meanwhile, Long Context Models excel in understanding large texts, useful for complex analyses or understanding broad topics without external references.
Listen to the full episode with remarks and further comments!
Listen now
Could AI be applied to your business case?
Subscribe to the newsletter and learn how AI can solve business challenges.