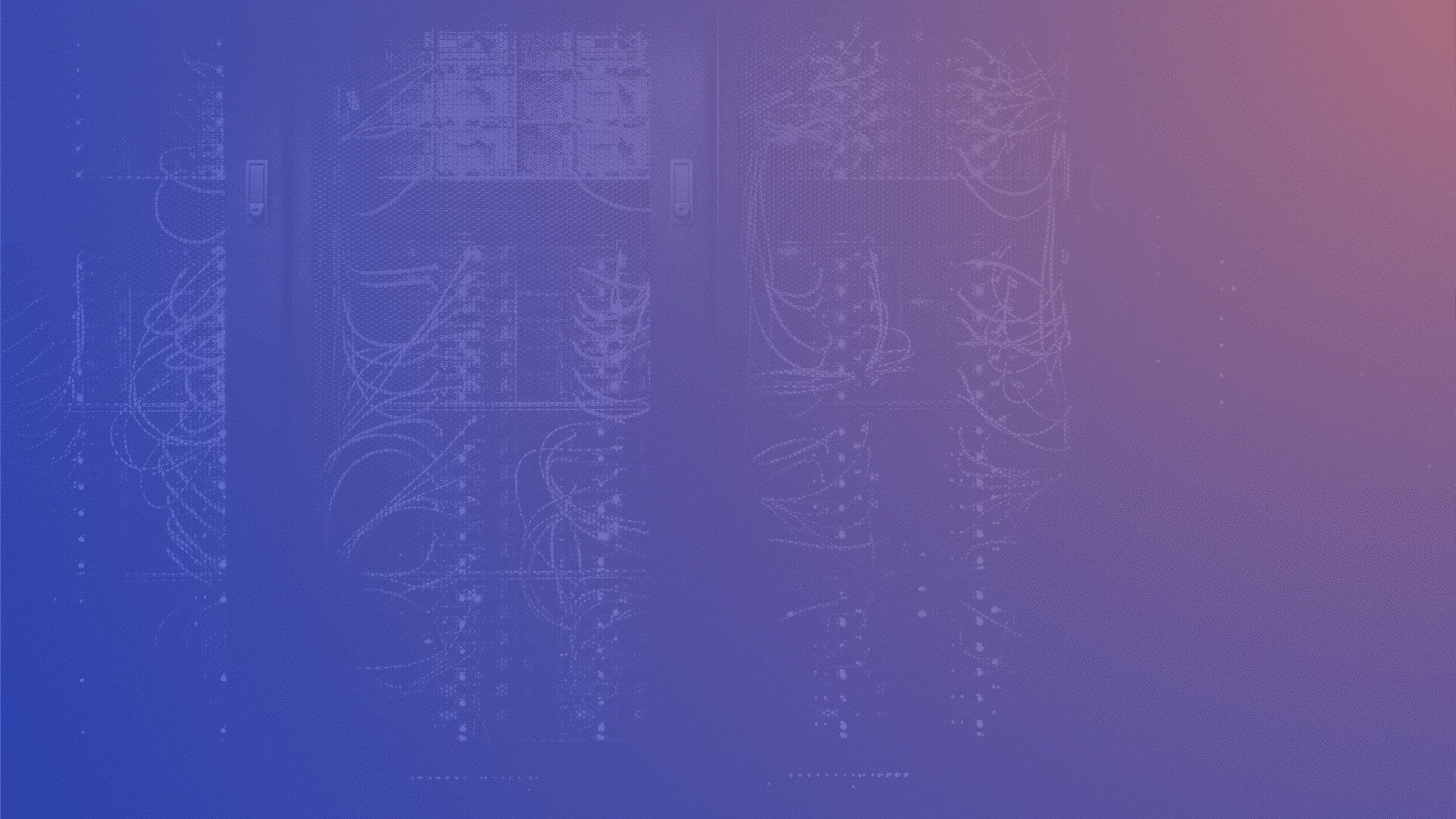
Have you been buying AI correctly?
Artificial intelligence as a concept is familiar to many, but as a technology, it is still quite abstract. Especially now there are many types of service providers, from technology consultants to information management professionals. Artificial intelligence is offered in many different forms – as ready-made products and applications or even as data models available for free – but increasingly artificial intelligence expertise is also purchased as a service.
However, there are many practical challenges associated with buying artificial intelligence as a service - what kind of data is needed, what precisely is the product I'm buying, can I do it myself or do I have to outsource? This blog serves as a first guide for you who are wondering "could this problem be solved with artificial intelligence - and can I do it myself or should I outsource?"
IDENTIFYING THE REAL PROBLEM - OR ASKING FOR HELP DEFINING THE PROBLEM
To start, it is good to define the business problem that you would like to solve with artificial intelligence. Although the applications of artificial intelligence might be hyped, it is not always suitable for every problem. Usually, AI works best in business processes where there is a lot of data. In such cases, data from different sources must be combined, and defining cause-and-effect relationships is difficult with just human input or traditional tools, but instead calculation and modeling is required. In this case, for example, machine learning methods are practically the only possible option. The same applies to machine vision: the more real-time or in-process footage there is, the easier it is to machine check it.
You don't necessarily have to do this brainstorming on your own – an AI agency can help you assess whether artificial intelligence, traditional statistics, advanced analytics - or a combination of several are better suited to the problem. Cooperation with an artificial intelligence agency often starts with mapping out different opportunities for utilizing artificial intelligence within the company or creating a plan for utilizing artificial intelligence together.
An external partner can be a great help already in the definition phase when you are wondering whether you need a consultant, a customized solution or ready-made software.
ENSURE DATA AVAILABILITY
When talking about artificial intelligence, the conversation quickly turns to available data. Contrary to popular belief, data does not always have to be available in huge quantities – it is more important that its availability is at the level required by the business, also when using models.
Let's have an example: an algorithm is being developed to predict the need for maintenance of a machine in a production line. Sensor data is easily available during the development phase from one place, where it has been collected from the machine regularly for years. When creating the algorithms it may be discovered that the sensor data is only obtained from the machine during its semi-annual maintenance. Usually data would be needed at least on a monthly basis, so that there would be some sense in giving a forecast of the maintenance need. This pitfall could be avoided by combining the sensor data of the production process with human observation data or the partner's process data with your own process data.
Regarding the amount of data, it is also decisive that there is enough of it about the variables - especially with the methods of traditional statistics. Of course, the most benefit from artificial intelligence is when large amounts of data can be processed automatically to solve a problem, but in addition to that, artificial intelligence solutions have a new kind of benefit: the "base" of the artificial intelligence algorithm can be the use of generally available large, right-oriented data sets, and the model can be "fine-tuned" with reasonably small amounts of just the right data.
For example, if you want to use machine vision to find certain types of cracks in the final product of the assembly line, freely available datasets of tens of thousands of images of various cracks can be used as the context of the machine vision algorithm. Finally, the model can be fine-tuned using a dataset of a few hundred images of the cracks found in the final products of this particular assembly line.
...And data ownership
Just as important as the availability of data is that it has an owner inside the house. When there is a lot of data scattered here and there and no one has a proper grasp of it, the progress of the project can be rocky.
It is fairly common to come across a situation where none inside the company knows the different variables or data measurement methods, and the data has been collected for 15 years with a collection script written by an employee who left the company ten years ago. In order to use artificial intelligence to solve the problem, it is therefore necessary to keep the ownership of the data up to date.
SET THE RIGHT METRICS FOR SUCCESS
Success can look different in all projects, but successful projects are characterized by the metrics agreed upon from the beginning and sticking to them. It is essential to set appropriate metrics for each project.
Let's have an other example: three mistakes out of ten thousand sounds computationally like great accuracy and thus a great result for an artificial intelligence model – but what if it's a model that identifies broken relays in a railroad rail traffic guidance system? Then it would be of utmost importance that the tolerance for error would be zero.
When buying artificial intelligence as a service, a skilled service provider will help you find business-relevant metrics and at the beginning of the project asses how likely the artificial intelligence solution will be able to meet them.
DEMAND A SOLUTION THAT SCALES YOUR – NOT THE SELLER'S – BUSINESS
Often offers are a one-size-fits-all solution, such as a ready-made solution or a product made to tackle several different artificial intelligence puzzles. A ready-made model may seem like a quick and cost-effective solution but it often supports scaling the seller's business more than the buyer's.
A tailored artificial intelligence solution, on the other hand, answers exactly the business problem of your company - and it doesn't always mean a bigger price tag. When you buy artificial intelligence as a service and not as a finished product, you can, for example, ensure that the seller of the service works with a hybrid model, i.e. uses software that is already in use within your company. This way, the solution is easier to integrate into the company's current processes and smarter to scale also within the company.
You should also be careful with IPR, because in terms of scaling the solution, it is important that you own the end result, i.e. the artificial intelligence solution yourself.
But shouldn't I do it myself?
More and more companies have their own data science team and also strong artificial intelligence expertise in-house. Therefore, at some point in the project planning or purchasing process the question often arises, isn't it worth doing it yourself?
Artificial intelligence should be purchased as a service when:
- You want to scale the utilization of artificial intelligence quickly - i.e. apply the same type of artificial intelligence-based service or methodology to several different business problems
- You want to make sure that the tools and methods you use are scalable - i.e. put the data management in order and make sure that the models run in the cloud efficiently, reliably and with continuous retraining - all this completely automatically, without large monitoring needs
- You want to adopt best practices and learn how others work
- Your own resources are already tied up in projects, but at the same time you should do experiments to open new projects
If you end up using an external partner, you should choose an vendor who implements artificial intelligence projects using a hybrid model together with the organization's R&D, IT or data science department and utilizes the company's existing systems as much as possible. This is how the team develops, important lessons remain in-house and it is easier to use them in other teams as well.
In many projects the hybrid model is a necessity: it would be expensive to build a team that would be taught everything from the beginning. On the other hand, if the team consists of partly the your own personnel, the experience can be harnessed quickly. This is often the case projects where we are creating a front end, an integration or a cloud solution. If implementing the project with a hybrid model is not possible for one reason or another, it is worth choosing a supplier that manages the project "end-to-end".
More resources
Could AI be applied to your business case?
Subscribe to the newsletter and learn how AI can solve business challenges.